【文章摘要】High-resolution remote sensing data enables the extraction of fine-detailed boundaries of open-pit minefields, which is crucial for various applications such as ecological restoration, environment impact assessment, mining field disaster minoring, etc. In the last decade, a variety of convolutional neural networks (CNNs) and vision transformers (ViT) approaches have been developed for extracting the boundary and coverage of open-pit minefields. However, these deep learning approaches are always computationally expensive in pretraining and fine-tuning the network parameters. In addition, the diverse land cover/land use of different open-pit minefields poses a big challenge in building a large-scale benchmark dataset. To conduct efficient open-pit minefield extraction with limited labelled data, this paper employs a large-scale foundation model called Segment Anything (SAM) to develop the few-shot learning strategy for extracting open-pit minefield with slightly fine-tuning SAM and without fine-tuning SAM, respectively. The experiment demonstrates that the proposed SAM-enhanced few-shot learning outperforms pretraining the-state-of-the-art semantic segmentation approaches in terms of extraction precision and time cost. We hope our work can provide a solution for complex open-pit minefield extraction with a small number of labelled datasets.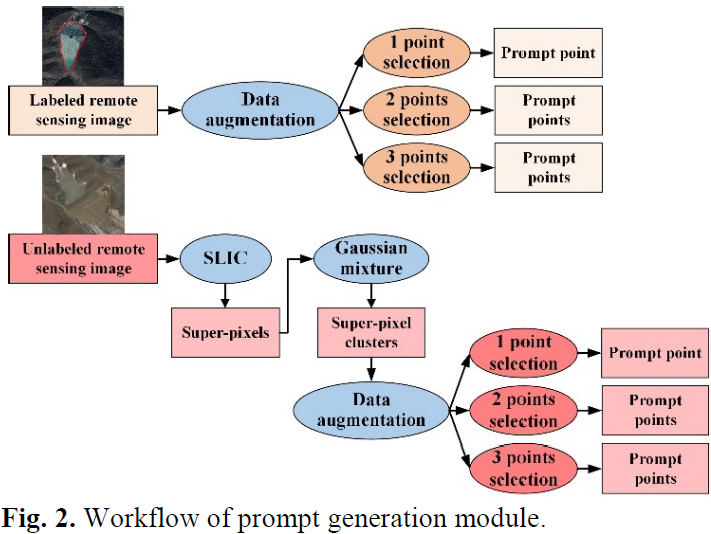
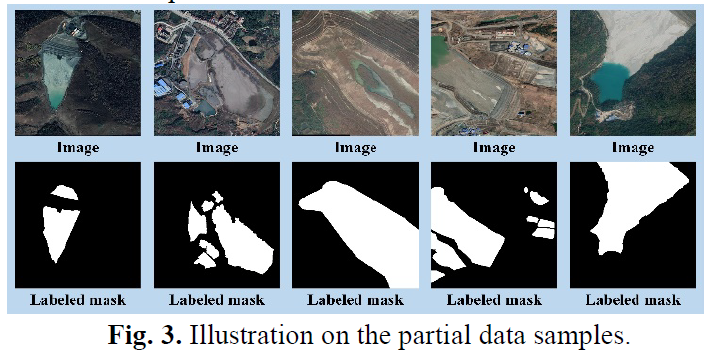
【文章信息】M. Shao, K. Li, Y. Wen and X. Xie, Large-Scale Foundation Model Enhanced Few-Shot Learning for Open-Pit Minefield Extraction, IEEE Geoscience and Remote Sensing Letters, vol. 21, pp. 1-5, 2024, Art no. 3003105. DOI: 10.1109/LGRS.2023.3342215.
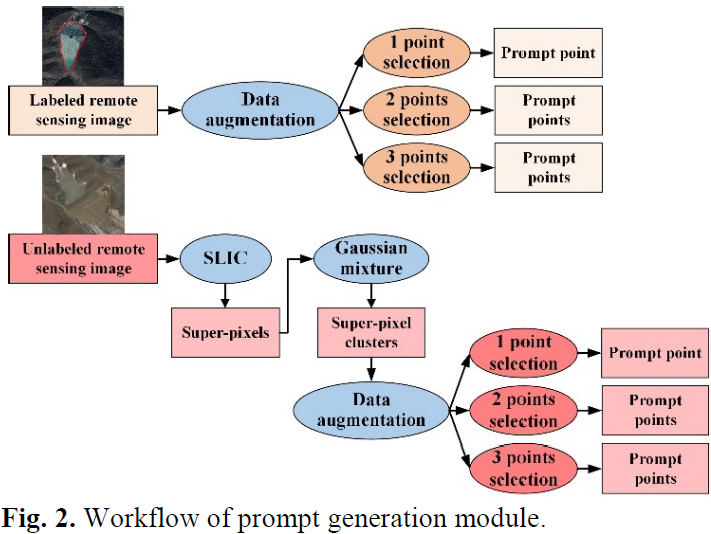
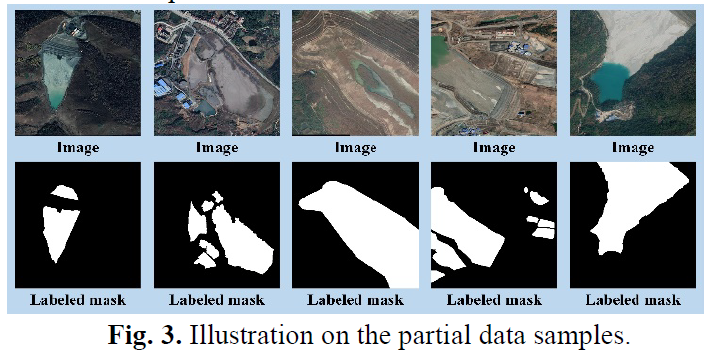